AI Artificial Intelligence Image Recognition Market Size, Share and Growth Report 2023 2030
Typically lesion detection algorithms need to be provided with annotations of a bounding box type usually encasing the lesion, while for training automatic segmentation models, radiologists need to outline lesions manually in multiple image slices121. Machine learning has a potent ability to recognize or match patterns that are seen in data. With supervised learning, we use clean well-labeled training data to teach a computer to categorize inputs into a set number of identified classes. The algorithm is shown many data points, and uses that labeled data to train a neural network to classify data into those categories. The system is making neural connections between these images and it is repeatedly shown images and the goal is to eventually get the computer to recognize what is in the image based on training. Of course, these recognition systems are highly dependent on having good quality, well-labeled data that is representative of the sort of data that the resultant model will be exposed to in the real world.
The Jump Start Solutions are designed to be deployed and explored from the Google Cloud Console with packaged resources. They are built on Terraform, a tool for building, changing, and versioning infrastructure safely and efficiently, which can be modified as needed. While these solutions are not production-ready, they include examples, patterns, and recommended Google Cloud tools for designing your own architecture for AI/ML image-processing needs. During the AI image recognition of the power supply equipment for determining the position of the switch, it is first necessary to determine the true position of the switch and manually observe to eliminate general faults or provide maintenance. Meanwhile, the corresponding on and off signs shall be set up to identify the change of the switch position, that is, it can automatically determine whether the switch is on. A lighter version of TensorFlow, TensorFlow Lite (.TFLITE) is customarily designed to run machine learning applications on mobile and edge devices.
Other fields
During data organization, each image is categorized, and physical features are extracted. This stage – gathering, organizing, labeling, and annotating images – is critical for the performance of the computer vision models. According to Fortune Business Insights, the market size of global image recognition technology was valued at $23.8 billion in 2019. This figure is expected to skyrocket to $86.3 billion by 2027, growing at a 17.6% CAGR during the said period. It can detect and track objects, people or suspicious activity in real-time, enhancing security measures in public spaces, corporate buildings and airports in an effort to prevent incidents from happening. Image recognition is also helpful in shelf monitoring, inventory management and customer behavior analysis.
- When radiologists used a deep learning model for detection and management of pulmonary nodules, their performance improved and reading time was reduced40.
- Finally, we ran prediction on the image we copied to the folder and print out the result to the Command Line Interface.
- From healthcare to retail, from autonomous vehicles to social media, image recognition is making a significant impact.
- A technological development as powerful as this should be at the center of our attention.
- The ninth image in the bottom right shows that even the most challenging prompts – such as “A Pomeranian is sitting on the King’s throne wearing a crown.
InbuiltData is at the heart of this transformative journey, offering the data and models needed to make AI-powered image recognition solutions a reality. Whether you’re a healthcare provider aiming to diagnose diseases earlier or an e-commerce company seeking to provide better product recommendations, InbuiltData is your trusted partner. The combination of AI and ML in image processing has opened up new avenues for research and application, ranging from medical diagnostics to autonomous vehicles. The marriage of these technologies allows for a more adaptive, efficient, and accurate processing of visual data, fundamentally altering how we interact with and interpret images.
Chemical As A Service Market Insights: Navigating the Path to Remarkable Growth in 2031
ML algorithms are usually developed using a training dataset, refined using a validation dataset, and then tested for their performance in an independent test dataset, ideally from a different institution. Despite their differences, both image recognition & computer vision share some similarities as well, and it would be safe to say that image recognition is a subset of computer vision. It’s essential to understand that both these fields are heavily reliant on machine learning techniques, and they use existing models trained on labeled dataset to identify & detect objects within the image or video.
These methods were primarily rule-based, often requiring manual fine-tuning for specific tasks. However, the advent of machine learning, particularly deep learning, has revolutionized the domain, enabling more robust and versatile solutions. An open-source machine learning library, TensorFlow has become a star resource for compiling and executing complex machine learning models. The comprehensive framework is used for various applications like image classification and recognition, natural language processing (NLP), and document data extraction. It can be easily paired with other machine learning tools such as OpenCV to add more value to any machine learning project. Computer Vision is a branch in modern artificial intelligence that allows computers to identify or recognize patterns or objects in digital media including images & videos.
Face detection and analysis
The outlining of disease, or segmentation, is fundamental to many AI/ML and radiomics studies, and is necessary to derive quantitative tumour measurements including tumour diameters, as well as generating tumour contours for radiotherapy planning62,63,64. Registration of segmentations across time-series can also inform clinicians on how tumours are changing with treatment. Manual tracing of lesion borders can lead to high inter-reader variability65, which may be reduced with automatic disease segmentation using AI models. Although deep neural networks are powerful enough to segment lesions, it is recommended that the final AI segmentation result should be verified by an experienced radiologist.
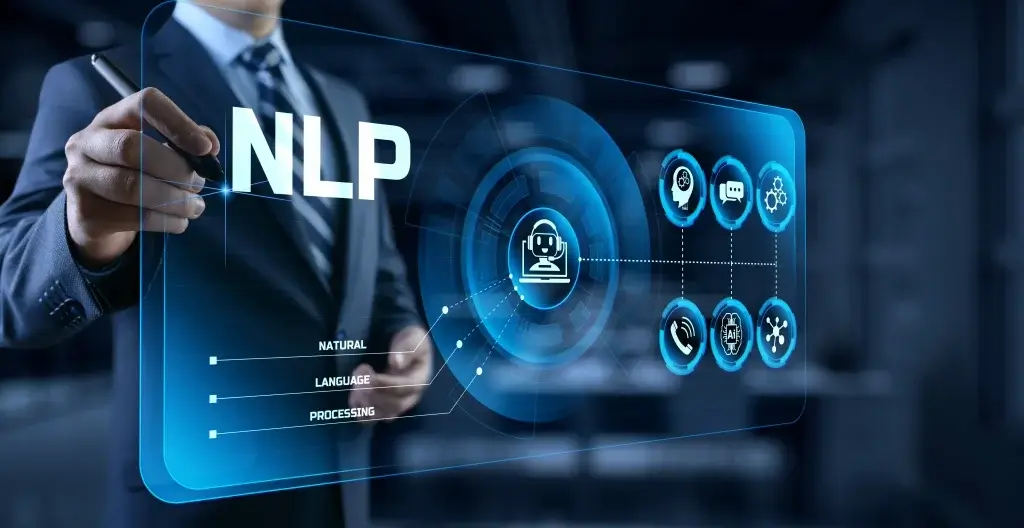
Read more about https://www.metadialog.com/ here.