5 Examples of AI Uses in Manufacturing The Motley Fool
A case study shows how manufacturing companies like Micron Technology have faced mechanical issues while developing their product. And how AI technology adoption has saved their hours of downtime and Avoided the loss of millions of USD through early detection of machine breakdowns and quality issues and a 10% increase in manufacturing output. AI-based cybersecurity software and risk detection can help in securing production factories. Manufacturers can use self-learning AI software to secure their IoT devices and cloud services. The system can also alert and provide guidance to prevent further damage. To avoid such scenarios, the manufacturers would schedule regular maintenance.
It leverages statistical models, AI, and a proprietary thread-based approach built on MITRE’s threat-informed defense strategy. Besides, with more dynamic data compliance policies, AI automates data access and security management throughout the business infrastructure. This allows companies to mitigate expensive legal suits due to data theft or sensitive data leaks.
Products and services
In 2003, Automation Anywhere, headquartered in San Jose, US, created a digital platform that integrates RPA with business processes to automate and analyze them. A bot created by Automation Anywhere will automate business processes and increase productivity by three times. Many manufacturers are still trying to adopt AI and ML-like modern technologies to reduce production costs and increase time-to-market. Following are some of the solutions of AI and ML that most manufacturers have adopted.
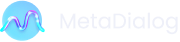
In 2017, Siemens developed a two-armed robot that can manufacture products without being programmed. There are AI solutions for manufacturing that can create more efficient systems to help reduce energy use on the production line. Imagine a world where manufacturing processes synchronize with seamless precision.
Improving manufacturing processes
Through this, energy companies are able to analyze historic energy consumption patterns and forecast future demands. Integration of the Internet of Things (IoT) and big data into production floors requires manufacturers to leverage AI. Otherwise, the digital touchpoints these technologies create go unutilized. AI-driven solutions in the manufacturing sector facilitate industrial automation, process monitoring, and production optimization. Retail companies primarily utilize AI to enhance customer experience through personalized product offerings and assisted in-store journeys. At the same time, the technology improves retail operations through predictive inventory planning and stock allocation.
- No, Definitely not,we have AI for that, and more specifically machine learning!
- Machine Learning algorithms are used to measure patterns in large datasets to inform more efficient decision-making processes.
- First, it can serve research purposes, allowing the companies to come up with new materials that carry desirable properties while being biodegradable or fully recyclable.
- Artificial intelligence and machine learning algorithms are used to derive insights from manufacturing data into product quality or predictions about product failures farther down in the production process.
- It’s clear that by investing in AI-driven technologies now will give companies an edge over competitors who have not yet adopted these solutions or even know about them.
Industrial robots, often known as production robots, automate monotonous operations, eliminate or drastically reduce human error, and refocus human workers’ attention on more profitable parts of the business. Preventive maintenance is another benefit of artificial intelligence in manufacturing. You may spot problems before they arise and ensure that production won’t have to stop due to equipment failure when the AI platform can predict which components need to be updated before an outage occurs. Manufacturers can use digital twins before a product’s physical counterpart is manufactured.
Inventory management
They can achieve that goal through efficient material treatment on the production line, as well as downtime reduction with preventive maintenance described above. That’s because a big part of industrial waste is the low-quality products not suitable for the market use, and downtimes can contribute to periodical quality decrease. So can the defects in machinery or the production process, easily detected by artificial intelligence. It operates in two segments, namely, Architecture & Software and Control Products & Solutions. Transitioning to a smart and clean grid is forcing the energy industry to rely on advanced data processing systems to aid load and demand-side management. Consequently, the energy generation and distribution markets see investments in advancing AI integration.
Although predictive quality analytics in manufacturing and predictive maintenance are often lumped into the same category, there are important differences between them. The premise of predictive maintenance is to use data from the production line to anticipate when manufacturing equipment is likely to fail, and then intervene to repair or replace the equipment before that happens. Moreover, were not dealing with some static one-time solution to a single specific problem but rather an ongoing quality optimization process that is based on multiple historical data.
A case study shows how Automation Anywhere’s RPA solution named Synergy helped automate billing processes can generate 163% of ROI. The IFR (International Federation of Robotics) report shows that there are 2.7 million robots currently operating in factories worldwide. McKinsey conducted a survey which results that the 4IR technologies are capable of generating approx. AI has the potential to generate $1.2-$2 trillion in value only in manufacturing.
Trending topics Q&A: digitalization – Aerospace Manufacturing and Design
Trending topics Q&A: digitalization.
Posted: Wed, 25 Oct 2023 02:54:02 GMT [source]
The way we observe objects and flaws is biased and many things may be different than they seem. With vast amounts of data on how products are tested and how they perform, artificial intelligence can identify the areas that need to be given more attention in tests. Though many believe personal, autonomous vehicles are the future, there are multiple ways in which AI and machine learning are being implemented in how vehicles are built and how they operate on the road. AI in cars aims to improve vehicle safety, increase fuel efficiency and provide drivers with enhanced connectivity features.
Additionally, AI enhances the use of electronic health record (EHR) data to identify trends and personalize healthcare delivery. This allows hospitals and doctors to ensure timely interventions and reduce patient risks. Based on the Innovation Map, the Tree Map below illustrates the top applications of artificial intelligence across 10 industries in 2023 and 2024. As AI aids data-driven decision-making, it greatly impacts the healthcare, retail, logistics, and energy industries. It collects and analyzes the data from sensors, electronic records, and other data management systems to identify patterns and trends to optimize operations. Some flaws in products are too small to be noticed with the naked eye, even if the inspector is very experienced.
AI systems can also take into account data from weather forecasts, as well as other disruptions to usual shipping patterns to find alternate route and make new plans that won’t disrupt normal business operations. BMW (BMWYY -0.23%) for example, uses AI to predict demand and optimize inventory. In one example, the company installed an AI application to prevent the transportation of empty containers on conveyor belts.
Quality Control and Defect Detection
These algorithms then make thousands of trades at a blistering pace with the goal of selling a few for small profits. Selling off thousands of trades could scare investors into doing the same thing, leading to sudden crashes and extreme market volatility. Widening socioeconomic inequality sparked by AI-driven job loss is another cause for concern, revealing the class biases of how AI is applied. Blue-collar workers who perform more manual, repetitive tasks have experienced wage declines as high as 70 percent because of automation. Meanwhile, white-collar workers have remained largely untouched, with some even enjoying higher wages.
In manufacturing, it can be effective at making things, as well as making them better and cheaper. The manufacturing industry has always been eager to embrace new technologies – and doing so successfully. Now, with AI adoption, they are able to make rapid, data-driven decisions, optimize manufacturing processes, minimize operational costs, and improve the way they serve their customers. This doesn’t mean that manufacturing will be taken over by the machines – AI is now an augmentation to human work and nothing can be a substitute of human intelligence and the ability to adapt to unexpected changes. Recommendation engines, for example, are used by e-commerce, social media and news organizations to suggest content based on a customer’s past behavior. Machine learning algorithms and machine vision are a critical component of self-driving cars, helping them navigate the roads safely.
Global Chip Race: Everything Investors Need to Know – Nasdaq
Global Chip Race: Everything Investors Need to Know.
Posted: Thu, 26 Oct 2023 17:01:21 GMT [source]
It can get used to automating complex tasks and trying different manufacturing patterns for a fast workflow. With an increasing emphasis on sustainable production on worldwide markets, waste reduction is becoming one of the manufacturers’ priorities – and artificial intelligence is irreplaceable in this field. Such solutions allow pharma companies to identify otherwise hidden target molecules or subtypes, speeding up drug discovery and development. Other applications of AI in pharma also include clinical trial risk assessment and precision medicine. Artificial intelligence has been creeping its way into the field, enabling self-driving vehicles to fulfill delivery orders.
By automatically creating optimized cutting plans (nesting) using AI that consider material utilization and the complexity of kitting operations, manufacturing efficiency can reach a new level. In fact, at its core AI is the process of building smart software capable of performing tasks that typically require human intelligence. Fueled by the massive amount of research by companies, universities and governments around the globe, machine learning is a rapidly moving target. Breakthroughs in AI and ML seem to happen daily, rendering accepted practices obsolete almost as soon as they’re accepted. One thing that can be said with certainty about the future of machine learning is that it will continue to play a central role in the 21st century, transforming how work gets done and the way we live. The goal is to convert the group’s knowledge of the business problem and project objectives into a suitable problem definition for machine learning.
- Lastly, AI-powered in-store analytics provides shelf intelligence that allows retailers to increase visibility into stocks and identify underperforming products.
- AI is being used inside many manufacturing operations to streamline processes and improve productivity.
- Now, with AI adoption, they are able to make rapid, data-driven decisions, optimize manufacturing processes, minimize operational costs, and improve the way they serve their customers.
- By using web-based RPA, users can automate any process using their browser.
- AI systems help manufacturers forecast when or if functional equipment will fail so its maintenance and repair can be scheduled before the failure occurs.
Read more about https://www.metadialog.com/ here.